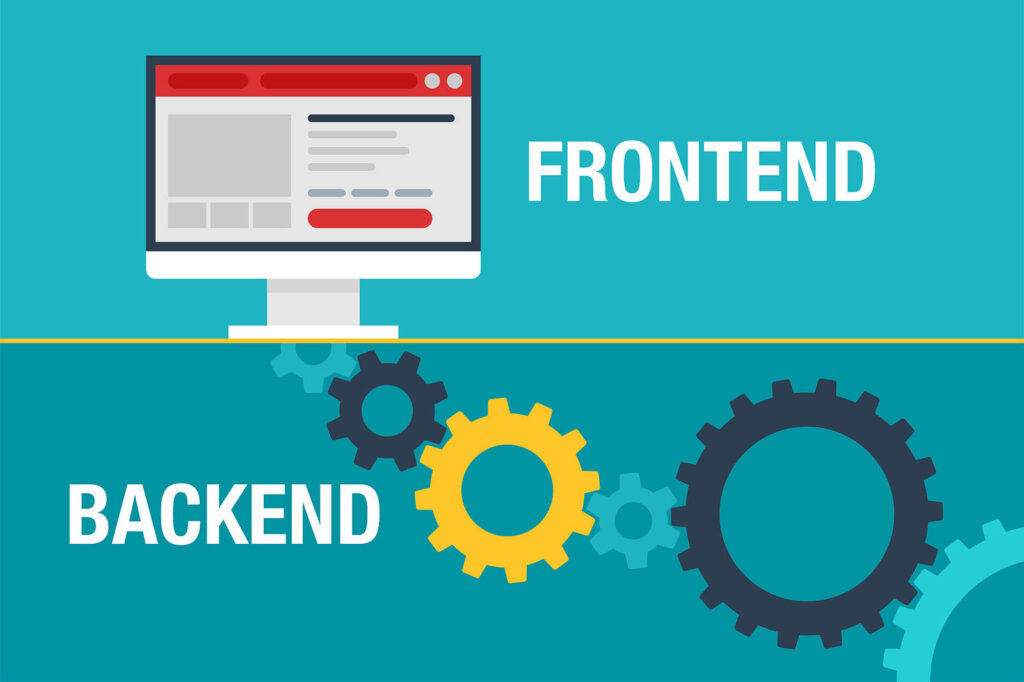
Computer simulations aid scientists in gauging battery performance
An important but not studied parameter that determines battery performance is a migration barrier. This determines the moving ion rate through the electrode in the battery, and in the end the charging or release rate. Because it is difficult to measure migration barriers in the laboratory, researchers usually use different computer simulations or estimates to quickly predict migration barrier values. However, very few of this simulation have been verified experimentally so far.
In a new study, researchers at the Indian Institute of Science (IISC) and their collaborators comprehensively analyze computing techniques that are widely used, and verify their predictions of migration barrier values to the actual data observed in laboratory measurements. Based on their analysis, the team proposed a series of strong guidelines to help researchers choose the most accurate computing framework for testing materials that can be used to develop very efficient batteries in the future.
Lithium-ion batteries, which give mobile and laptop power, consist of three main components: dense negative electrodes (anode), solid positive electrodes (cathodes) and liquid or solid electrolytes that separate them. When filling or releasing, lithium ions migrate across electrolytes, creating potential differences. “Electrodes in lithium-ion batteries are not 100% solid. Think like a sponge. They have ‘pores’ through which lithium ions,” explained Sai Gautam Gopalakrishnan, assistant professor in the Department of Material Engineering, IISC, and the appropriate authors from the paper Published in NPJ Computational Material.
Important parameters that determine the rate at which lithium ions penetrate the pores are migration barriers – energy thresholds that need to be overcome by ions to cross electrodes. “The lower the migration barrier, the faster you can fill or remove the battery,” said Reshma Devi, Ph.D. Students in the Department of Material Engineering and the first research authors.
“The same migration barrier value is calculated by one group using one computing technique and another group using another technique. The values may be equal, but we cannot know that with certainty,” explained Gopalakrishnan.
Two specific estimates, which are called highly limited and normally normal (scan) and general gradient estimates (GGA), are the most widely used methods for computing up to migration barriers, but each has its own losses. “We took nine different ingredients,” Reshma Devi explained. “We examine which estimates are closest to the experimental value for each.”
The team found that the functional scanning has a better numerical accuracy as a whole, but the GGA calculation is faster. GGA is found to have a reasonable level of accuracy in calculating migration barriers in certain ingredients (such as lithium phosphate), and may be a better choice if rapid estimates are needed, the researcher’s advice.
Such insight can be valuable for scientists who are trying to test new material for their performance before they are adapted for battery -related applications, said Gopalakrishnan. “Suppose you have material that is unknown and if you quickly want to see if this material is useful in your application, then you can use calculations to do that, as long as you know which computing estimates give you the closest value. This is useful when coming for the discovery material. “
The team also works to develop machine learning tools that can help accelerate the prediction of migration barriers for various materials.